Hypothesis testing is a necessary procedure in statistics. A hypothesis test evaluates two mutually exclusive statements to determine which statement is best supported by sample data. When it is said that a find is statistically significant, this is due to testing the hypothesis.
Verification Methods
Statistical hypothesis testing methods are statistical analysis methods. Typically, two sets of statistics are compared, or the data set obtained by sampling is compared with a synthetic data set from an idealized model. Data should be interpreted in a way that adds new meanings. You can interpret them, assuming a certain structure of the final result and using statistical methods to confirm or reject the assumption. An assumption is called a hypothesis, and the statistical tests used for this purpose are called statistical hypotheses.
Hypotheses H0 and H1
There are two basic concepts of statistical hypothesis testing - the so-called “basic or null hypothesis” and “alternative hypothesis”. They are also called Neumann-Pearson hypotheses. The assumption of a statistical test is called the null hypothesis, the main hypothesis, or H0 for short. It is often called the default assumption or the assumption that nothing has changed. Failure to test assumptions is often referred to as the first hypothesis, alternative hypothesis, or H1. H1 is an abbreviation for some other hypothesis, because all that is known about it is that data H0 can be discarded.
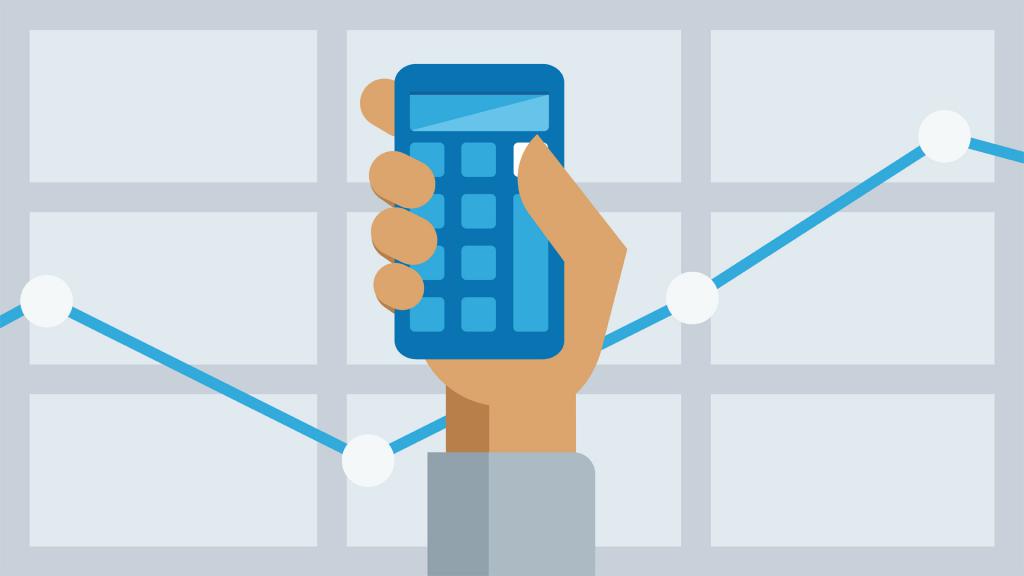
Before rejecting or not rejecting the null hypothesis, it is necessary to interpret the test result. A comparison is considered statistically significant if the relationship between the data sets is an unlikely realization of the null hypothesis in accordance with a threshold probability - significance level. There are also criteria for agreement on statistical hypothesis testing. This is the name of the hypothesis test criterion, which is associated with the proposed law of unknown distribution. This is a numerical measure of the discrepancy between the empirical and theoretical distribution.
Procedure and criteria for testing statistical hypotheses
The most common hypothesis selection methods are based on either the Akaike information criteria or the Bayes coefficient. Statistical hypothesis testing is a key method for both inference and Bayesian inference, although the two types have notable differences. Statistical hypothesis tests define a procedure that controls the likelihood of an erroneous decision being made about an incorrect hypothesis by default or a null hypothesis. The procedure is based on how likely it is that it will work. This probability of making a wrong decision is the improbability that the null hypothesis is true and that no specific alternative hypothesis exists. The test cannot show its truth or falsehood.
Alternative Decision Making Methods
There are alternative methods of decision theory in which the null and first hypotheses are considered on a more equal basis. Other decision-making approaches, such as Bayesian theory, try to balance the consequences of wrong decisions in every way, rather than concentrating on one null hypothesis. A number of other approaches to deciding which hypothesis is correct are based on data which of them have desirable properties. But hypothesis testing is the dominant approach to data analysis in many fields of science.
Testing the statistical hypothesis
Whenever a set of results differs from another set, one must rely on testing statistical hypotheses or statistical tests of hypotheses. Their interpretation requires a correct understanding of p-values and critical values. It is also important to understand that, regardless of significance level, tests may still contain errors. Therefore, the conclusion may be wrong.
The testing process consists of several stages:
- An initial hypothesis is being created for the study.
- The corresponding null and alternative hypotheses are indicated.
- Statistical assumptions about the sample during the test are considered.
- It is determined which test is suitable.
- The significance level and the probability threshold are chosen below which the null hypothesis will be rejected.
- The distribution of test statistics for the null hypothesis shows the possible values at which the null hypothesis is rejected.
- The calculations are carried out.
- The decision is made to reject or accept the null hypothesis in favor of the alternative.
There is an alternative where the p-value is used.
Significance Criteria
Pure data is not practical without interpretation. In statistics, when you need to start asking questions about data and interpreting the results, statistical methods are used that ensure the accuracy or probability of answers. When testing statistical hypotheses, this class of methods is called statistical testing, or significance criteria. The term “hypothesis” recalls scientific methods, where hypotheses and theories are studied. In statistics, a hypothesis test as a result produces a certain quantitative value under a given assumption. It allows one to interpret whether an assumption is being fulfilled or whether a violation has been committed.
Statistical interpretation of tests
Hypothesis tests are used to determine which research results will result in the rejection of the null hypothesis for a predetermined significance level. The test results of the statistical hypothesis must be interpreted in order to continue working on it. There are two common forms of criteria for testing statistical hypotheses. These are p-value and critical values. Depending on the selected criterion, the results obtained need to be interpreted in different ways.
What is p value
The conclusion is described as statistically significant in interpreting the p-value. In fact, this indicator means the probability of error if the null hypothesis is rejected. In other words, one can name the value that can be used to interpret or quantify the test result and determine the probability of error when the null hypothesis is rejected. For example, you can perform a normal distribution test in a data sample and detect the improbability of the deviation. Moreover, there is no need to abandon the null hypothesis. A statistical hypothesis test may return a p-value. This is done by comparing the value of p with a predetermined threshold value called the significance level.
Significance level
The significance level is often written in the Greek lowercase letter "alpha". The total value used for alpha is 5%, or 0.05. A smaller alpha value implies a more reliable interpretation of the null hypothesis. The p-value is compared with a pre-selected alpha value. The result is statistically significant if the p-value is less than alpha. The significance level can be inverted by subtracting it from unity. This is done to determine the level of confidence of the hypothesis, given the observed sample data. Using this method of testing statistical hypotheses, the P-value is probabilistic. This means that in the process of interpreting the result of a statistical test, it is not known what is true or false.
Theory of testing statistical hypotheses
Rejection of the null hypothesis means that there is enough statistical data on whether it looks likely. Otherwise, this means that there is not enough statistics to reject it. We can talk about statistical tests in terms of the dichotomy of deviation and the adoption of the null hypothesis. The danger of the statistical criterion for testing the null hypothesis is that, if adopted, it may seem that it is true. Instead, it would be more correct to say that the null hypothesis is not rejected, since there are not enough statistics to reject it.
This point is often confusing for beginner extras. In such a case, it is important to remind yourself that the result is probabilistic and that even the adoption of the null hypothesis still has a low probability of an error.
True or false null hypothesis
The interpretation of p does not mean that the null hypothesis is true or false. This means that a choice was made to reject or not reject the null hypothesis at a certain level of statistical significance based on empirical data and the chosen statistical test. Therefore, the p-value can be considered as the probability of the data presented in a pre-determined assumption embedded in statistical tests. The p-value is a measure of how likely it is that a data sample will be observed if the null hypothesis is true.
Interpretation of critical values
Some tests do not return p. Instead, they can return a list of critical values. The results of such a study are interpreted in a similar way. Instead of comparing one p-value of a predetermined significance level, test statistics are compared with a critical value. If it turns out to be smaller, this means that it was not possible to reject the null hypothesis. If greater than or equal, the null hypothesis should be rejected. The meaning of the algorithm for testing the statistical hypothesis and interpreting its result is similar to the p-value. The selected level of significance is a probabilistic decision to refuse or not reject the basic assumption of the test taking into account the data.
Statistical Test Errors
Interpretation of a test of a statistical hypothesis is probabilistic. The task of testing statistical hypotheses is not to find a true or false statement. Test evidence may be erroneous. For example, if alpha was 5%, this means that for the most part 1 out of 20 times the null hypothesis will be rejected by mistake. Or it will not be due to statistical noise in the data sample. Given this point, a small p-value at which the null hypothesis should be rejected may mean that it is false or that an error was made. If this type of mistake is made, the result is called false positive. And such a mistake is a mistake of the first kind when testing statistical hypotheses. On the other hand, if the value of p is large enough, which means rejecting the rejection of the null hypothesis, this may mean that it is true. Or not true, and some unlikely event occurred, due to which a mistake was made. This type of error is called false negative.
Error probability
When testing statistical hypotheses, there remains a chance to make any of these types of errors. False data or false conclusions are likely. Ideally, you must select a level of significance that minimizes the likelihood of one of these errors. For example, a statistical test of null hypotheses can have a very low level of significance. Although significance levels such as 0.05 and 0.01 are common in many fields of science, the most common use is a significance level of 3 * 10 ^ -7, or 0.0000003. It is often called “5-sigma”. This means that the conclusion was random with a probability of 1 in 3.5 million independent repetitions of experiments. Examples of testing statistical hypotheses often carry similar errors. This is also the reason why it is important to have independent verification results.
Statistical Verification Examples
There are several common examples of putting hypothesis testing into practice. One of the most popular is known as “Tea Tastings”. Dr. Muriel Bristol, a colleague of the founder of biometrics Robert Fischer, claimed that he could tell for sure whether tea or milk was the first to be added to the cup. Fisher suggested giving her eight cups (four from each variety) in random order. The test statistics were simple: counting the number of successes in choosing a cup. The critical region was the only success case out of 4, possibly based on the usual probability criterion (<5%; 1 out of 70 ≈ 1.4%). Fisher argued that an alternative hypothesis was not required. The lady correctly identified each cup, which was considered as a statistically significant result. Thanks to this experience, Fisher’s book, Statistical Methods for Researchers, appeared.
Example with the defendant
The statistical test procedure is comparable to a criminal court, where the defendant is presumed innocent until proven guilty. The prosecutor is trying to prove the defendant’s guilt. Only when there is sufficient evidence for the prosecution can the defendant be convicted. At the beginning of the procedure, there are two hypotheses: "The defendant is not guilty" and "The defendant is guilty." The hypothesis of innocence can be rejected only when the error is very unlikely, because one does not want to condemn the innocent defendant. Such a mistake is called a mistake of the first kind, and its occurrence is rarely controlled. As a consequence of this asymmetric behavior, a mistake of the second kind, that is, the acquittal of the person who committed the crime, is more common.
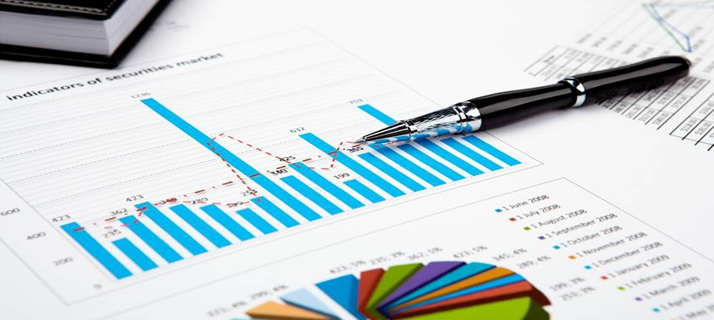
Statistics are useful in analyzing large amounts of data. This applies equally to testing hypotheses that can justify conclusions, even if no scientific theory exists. In the tea tasting example, it was “obvious” that there is no difference between whether milk is poured into tea or whether tea is poured into milk.
A real practical hypothesis testing application includes:
- testing whether men more than women suffer from nightmares;
- authorship of documents;
- assessment of the effect of the full moon on behavior;
- determining the range in which a bat can detect an insect using an echo;
- the choice of the best means to quit smoking;
- checking whether the bumper stickers reflect the behavior of the car owner.
Statistical hypothesis testing plays an important role overall in statistics and in statistical conclusions. Testing the value is used as a replacement for the traditional comparison of the predicted value and the experimental result in the core of the scientific method. When a theory can only predict the sign of a relationship, directed hypothesis tests can be configured in such a way that only a statistically significant result supports the theory. This form of valuation theory is the most severe criticism of the application of hypothesis testing.